AI Training Challenges and Solutions: Navigating the Path to Optimal Model Training
Oct 12, 2023
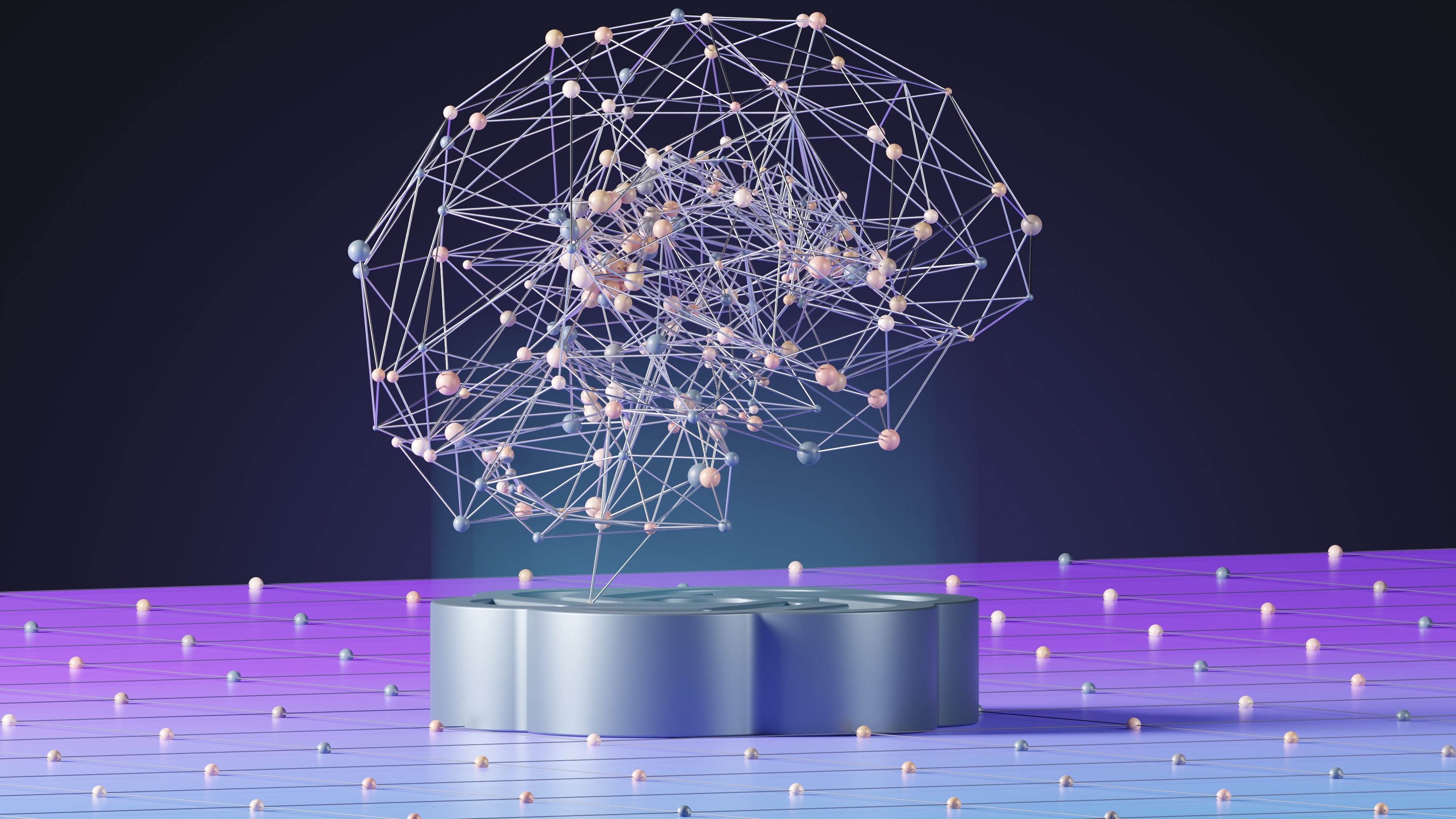
In the ever-evolving landscape of artificial intelligence, the journey from concept to a well-trained model is often marked by challenges that can significantly impact the model's performance and integrity. This blog post explores common hurdles encountered during AI model training.
Challenge 1: Training Data Quality
One of the foremost challenges in AI model training is ensuring the quality of training data. Many popular data annotation providers fall short of meeting client expectations, resulting in compromised model outcomes. This inadequacy stems from a lack of comprehensive quality control measures.
NEAR Tasks recognizes the paramount importance of data quality. To address this challenge, the platform incorporates a robust quality review system. This system scrutinizes task outputs, combining both automated and human verification, to ensure consistency and accuracy.
Furthermore, NEAR Tasks introduces a tasker reputation, aligning the interests of taskers and task providers. This alignment drives better data quality, benefiting all stakeholders involved.
Challenge 2: Data Bias
Another significant challenge in AI training revolves around data bias. Many widely-used annotation providers rely on location-bound annotators, limiting the diversity and representativeness of the data. Consequently, models trained with such data tend to exhibit biases, as they do not capture the nuances of humanity as a whole.
NEAR Tasks takes a global approach to mitigate data bias. By tapping into a diverse pool of taskers worldwide, the platform ensures that data annotation is not confined to specific regions or demographics. This global reach minimizes biases inherent in location-bound data annotation, fostering more equitable and unbiased AI models.
Challenge 3: Cost of Data
The cost of data annotation is often a source of concern for AI model developers. While it's essential to compensate data annotators fairly for their time and effort, the focus should shift from the total cost to the cost per quality. Many clients find themselves in a situation where they need to hire additional personnel to manually review data provided by annotation providers. Often, this cost is not factored into the consideration of the provider's pricing.
NEAR Tasks approaches the cost of data annotation with a quality-driven mindset. By prioritizing data quality, the platform reduces the need for additional manual reviews, ultimately saving clients time and resources. NEAR Tasks' transparent pricing structure ensures that clients receive value for their investment by delivering high-quality annotated data.
Conclusion: A Brighter Future for AI Training
In the realm of AI model training, challenges are inevitable, but so are solutions. NEAR Tasks, with its commitment to data quality, global reach, and cost-effectiveness, is poised to redefine the AI training landscape. By addressing these common challenges head-on, NEAR Tasks empowers AI developers to embark on their training journey with confidence, knowing that their models will be built on the foundation of quality, diversity, and cost-efficiency.
As the AI industry continues to advance, the role of AI task platforms like NEAR Tasks becomes increasingly vital. By aligning interests, fostering inclusivity, and emphasizing data quality, NEAR Tasks contributes to a future where AI models are not only powerful but also ethical and equitable, ultimately benefiting society as a whole.
Web3 and the Future of AI: Bridging the Divide for a New Era of Work
Nov 12, 2023
The Rise of AI Task Marketplaces: Transforming the Gig Economy
Oct 30, 2023
The Importance of High-Quality Human Training Data in AI
Oct 26, 2023
How to Monetize Your Skills on AI Task Platforms: A Guide to Earning Income
Oct 20, 2023